Our mission is the development of robust process models tailored specifically for semiconductor manufacturing processes. These sophisticated models have the capability to accurately predict process outcomes without necessitating physical product measurements. By leveraging advanced technologies, we aim to optimize and control manufacturing processes in real-time, while effectively mitigating process drift and discrepancies between tools.
The intricacies of semiconductor manufacturing processes often render full-scale simulation models impractical, and pure AI-driven models frequently fall short in practical applications. Our approach addresses these challenges by integrating production data, simulations, empirical research findings, and the expertise of our process researchers. Through this interdisciplinary collaboration, we create highly reliable and resilient models and digital twins that can withstand real-world complexities.
Our research focus extends to implementing automatic analysis of metrology results by employing cutting-edge computer vision algorithms. Additionally, we utilize fast surrogate models to accelerate physical simulations, ensuring swift and accurate insights into process dynamics.
Research Topics
- Models for production data: Hybrid physics-informed AI, semi-supervised learning, and classical machine learning algorithms
- Computer vision: Image analysis for inline metrology
- Real-time: Fast surrogate models for long simulation runs
- Use cases: Chemical-mechanical planarization (CMP) and plasma etching
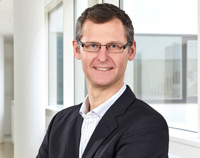
Recent Publications
Rothe, T., et al. (2023). Towards knowledge-enhanced process models for semiconductor fabrication. IITC/MAM
Zienert, A., et al. (2023). Automatic Detection of Via Arrays in AFM Images for CMP Dishing Evaluation. IITC/MAM
Abdin, A. (2021). Applying self-normalizing neural networks to tackle data-driven soft sensing problems in manufacturing lines. Big Data